Unusual SEO Analysis or “Redesign Impact on E-commerce SEO”

If you’re in analytics or product management where SEO plays an important role and is part of your customer acquisition strategy, then you’ve already wondered how to analyze search engine optimization performance less noisily and more effectively. How to make decisions based on data, or more importantly, how to make sure that some major changes have not led you to weaken this acquisition channel.
Let’s start with the background
The team and I have been planning a redesign of the site for a long time, which has not undergone significant changes for more than 10 years. And most of these changes to the product are not difficult to check and calculate — you can compare funnels, point-check the hypotheses previously formulated for the redesign, or even build paths based on user sessions (I will talk about this a little later in the future articles).
But what to do with evaluating the impact of such a massive change on SEO was not entirely clear.
Standard methods are not suitable for us due to the specifics of the product — we help sell tickets for events for which we often have exclusive rights. What makes us a product where a fairly large percentage of traffic is attracted to us by the organizer himself, whose fame also affects organic traffic, regardless of the position of our pages in the search.
In general, at first glance, the task seemed more trivial than it actually turned out :)
Need to talk to everyone
In order to understand the specifics of the issue and the task better, it is necessary to learn not just opinions, but rather the understanding and definitions of the parties.
I will not stretch this chapter and just collect bullet points what notes I managed to collect in the process of studying the issue:
- It would be wrong to classify different pages under the same brush or even combine them into groups for analysis by type — each page in our particular case is unique since all activities are different and different work is carried out on them (even despite the general redesign and change in the general page template );
- Behind the general redesign analytics, we cannot lose and blur separate work on SEO optimization for individual projects, since such a report will most likely be used not just as an assessment of the redesign, but as analytics of the general effectiveness of the SEO strategy. We need to help our team evaluate the redesign and, along with it, the SEO team evaluate their separate work;
- As I mentioned above, just traffic from the Search console cannot be a universal indicator for us, since it is affected by a lot of noise that we cannot clean out;
- Pages may disappear and appear. The listing of offers on our resource can be either permanent/recurrent or one-time deals. This must certainly be counted;
- The resource of the SEO team is limited, and a series of data can only be collected manually and this must be done regularly. The amount of data for manual collection should not affect the work of the guys and take away too many resources from them.
Search for approaches and tools
As we found out — the task is interesting! Let’s go poking around in the halls of the mind and google.
Everything that we managed to come up with at first did not fit on 1–2 points, and the satisfaction of each point for us in this task and under the conditions of the specifics of the product is important.
It’s like with bone marrow transplantation — all 5 points must match, otherwise, there will be an autoimmune reaction and the body will not accept what has just been transplanted into it. And in our case, the result of such an inaccurate study can be used as a coaster for a coffee mug, since it will be impossible to believe it at least 90%.
Below are the methods that I found, and their pros and cons specifically in our case (although I have to note, our case is far from unique to e-commerce, so this table may be relevant for you):

At the very last moment, and unexpectedly for myself, I found a non-standard application of the scatter plot in the context of this task.
Now let’s take a look at this interesting way to marry metrics.
It’s worth reiterating that a number of these work and apply to our product specification, but I’m sure these theses will apply to many e-commerce companies!
Scatter plots as an SEO analysis method
A scatter plot (also called a scatterplot, scatter graph, scatter chart, scattergram, or scatter diagram)[3] is a type of plot or mathematical diagram using Cartesian coordinates to display values for typically two variables for a set of data.
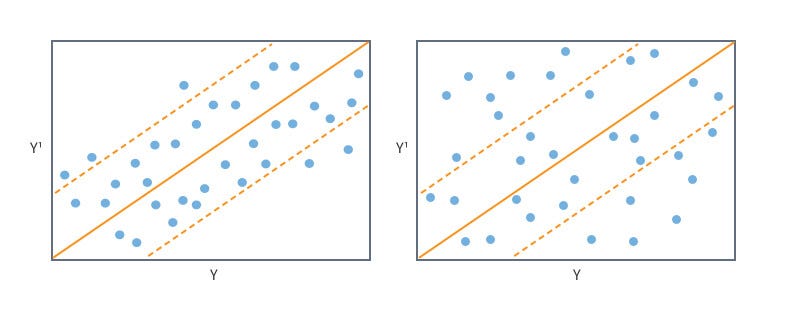
In our product, simple SEO analysis is not suitable for two main reasons (but not only for them):
- A high level or increase in organic traffic from SERPs can occur regardless of whether changes have been made. And may not even be related to the position of the page;
- Positions in search results may not bring traffic, despite all the possible types of steps taken toward SEO optimization. This may depend on the season and supply within the niche, and bring high results later.
At the same time, the scatter plot, where one dot is a page+request, will show the dependence of these two metrics. And the multi-colored visual of the series and the display of vectors by entities from one series to another will show the dynamics.
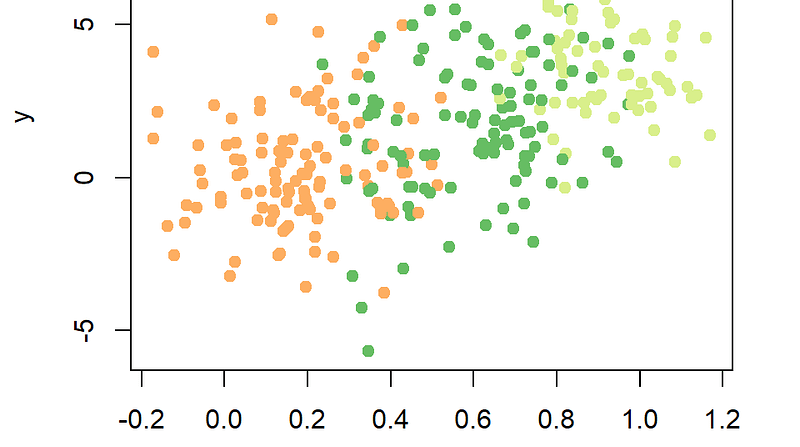
In the context of redesign, clouds (clusters) of such points and the migration of these clouds from series to series will show the effectiveness of mass changes, just such as redesign.
What data is needed and how to prepare it?
Step one
Collecting data about the positions of pages in the search and impressions on the selected search queries is necessary. This list should be compiled in advance, determining which pages will live longer and which will change.
Once you have managed to collect such a list, you need to go to SEO specialists and determine the number of search queries by which users find these pages in the search.
As a result, we get something like this sign:

That is, as you may have noticed, pages are not unique things. Several different requests can lead to each page, and the positions in the search and traffic to this page are highly dependent on requests.
Step two
We collect data about positions and impressions on these pages. We do this in series at equal intervals.
For example, a week is selected as an interval, which means that we place in one series of information about the average position of the page in the search for the week and the total volume of impressions that we collected with such a request on such a page. It turns out something like this:

Step three
We reduce the table into a format that accepts scatter charts from Google Sheets or ask analysts to do the same in python. I won’t go into detail because the final data format is very dependent on the charting tool you choose.
After the information is collected, we build a diagram. The X-axis will contain impressions, and the Y-axis will contain positions. The Y-axis, by the way, is better to expand so that the largest position is at the bottom, and the smallest one is at the top. This will later help us to read and analyze these diagrams more visually and logically. This is easy to do — you can make the page positions negative numbers with the same module (for example, 3 becomes -3, just for readability).
As a result, we should get something like this:
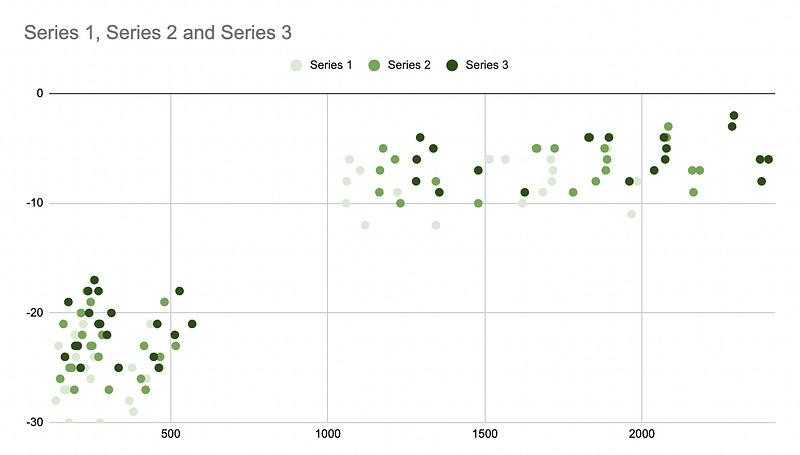
How to read it?
In fact, everything is very easy to read and analyze. Let’s point by point.
It is worth noting that this is a data sample, so there are no examples of how something got worse :) But by absolute analogy with the improvement patterns that I will show, deterioration patterns also work, only vectors (soon you will understand what I mean) will be directed in the other direction.
Impact of the entire redesign
We need to evaluate the impact of mass changes, and therefore the impact will be massive. Let me remind you that each point on the diagram is a request + page. Accordingly, if we change the template of a group of pages at once, then the changes on the chart should also occur as a group.
Below is the picture, I left only one series to easier understand where the groups are:
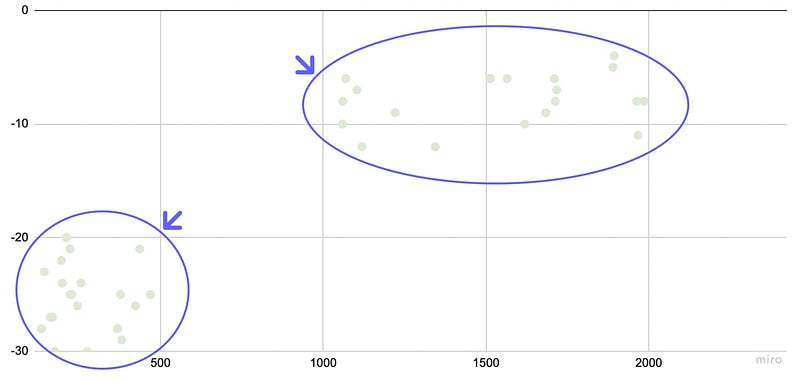
The graph clearly shows two clouds. Each of them is a group of pages. For example, product pages and category pages. They stand out in such isolated groups on the chart, because the traffic volumes and positions in the search for each group are different.
Let’s add the last series to see what happened to the clouds (groups of pages):
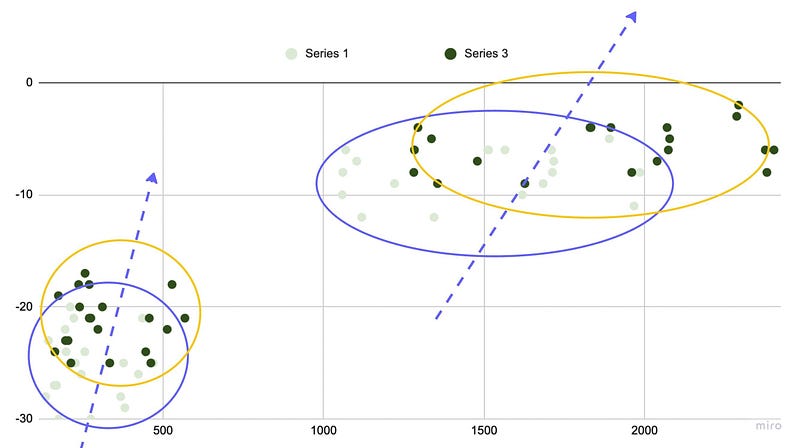
I highlighted the new series with yellow shapes to make it clearer and made the vectors. What do you see on this chart?
In fact, the graph shows very clearly how entire groups of pages went diagonally up in the new series, which means that the template changes for these types of pages were successful — they climbed higher in the search (vector up the Y axis) and depending from this received more traffic (to the right on the x-axis).
It’s worth noting that a ~45º upward consistent movement to the right indicates pure causation: Better SEO –> Higher search position –> More traffic.
Let’s take a look at special cases of the direction of vectors and, as a result, the conclusions that can be made for each shift in one direction or another:
- The clouds are not moving — it is obvious that nothing has changed in general. Probably, there are changes in special cases, but we will analyze this in the following sections of the article.

- The clouds moved to the right but did not move along the Y-axis. Such a move suggests that we did not globally influence the quality of page groups from the side of SEO, but began to organically receive more traffic. This is due to the increasing trend in the search and may indicate the start of the season, for example.
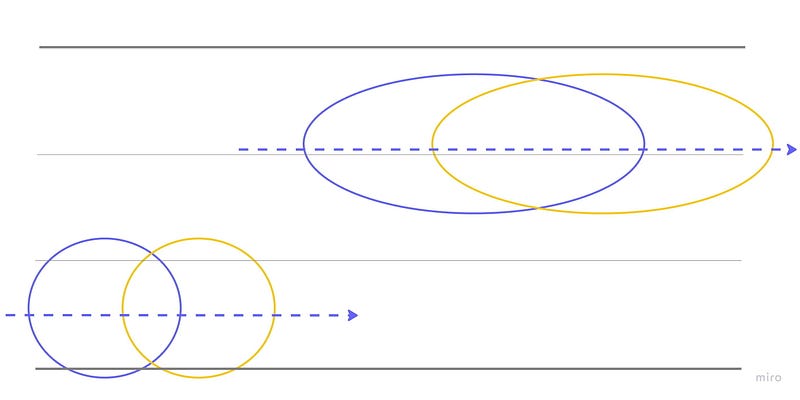
- The clouds went up but didn’t move on the x-axis. This behavior is common with SEO improvements that have resulted in an improvement but have yet to result in traffic. Easier: we are already at the top, but we are not yet / yet / generally searched for (it can also indicate incorrectly selected and used keywords on the page).

- Everything went left and down. There has been a massive downgrade.
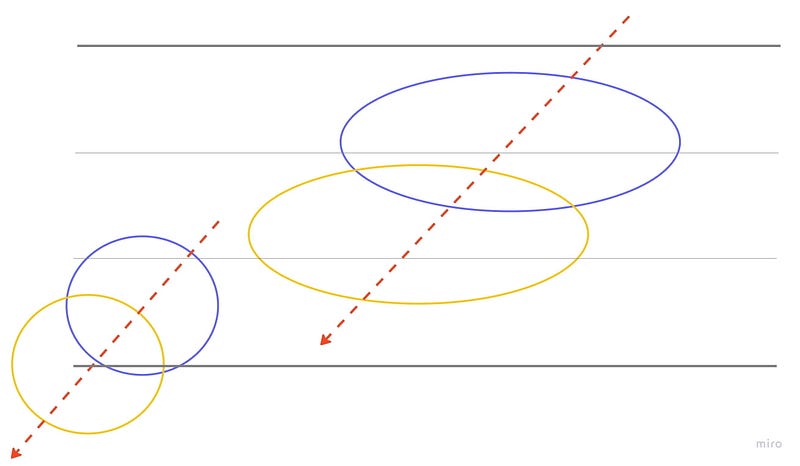
Targeted work of the SEO team
Let me remind you that one of the tasks that we pursued was not only to evaluate the effectiveness of mass changes to groups of pages but also to analyze the result of targeted work on individual pages on the platform.
What I mentioned earlier will help us with this — each dot on the scatter chart is literally a page + request.
That is, if:
- save each link page + request under its id in the analytics table
- link vectors old and new points related to the same pages
… then we will see the movement not only of groups but also of points that have strayed from the flock, which, for one reason or another, have become more effective than the main mass or less effective.
Let’s see:
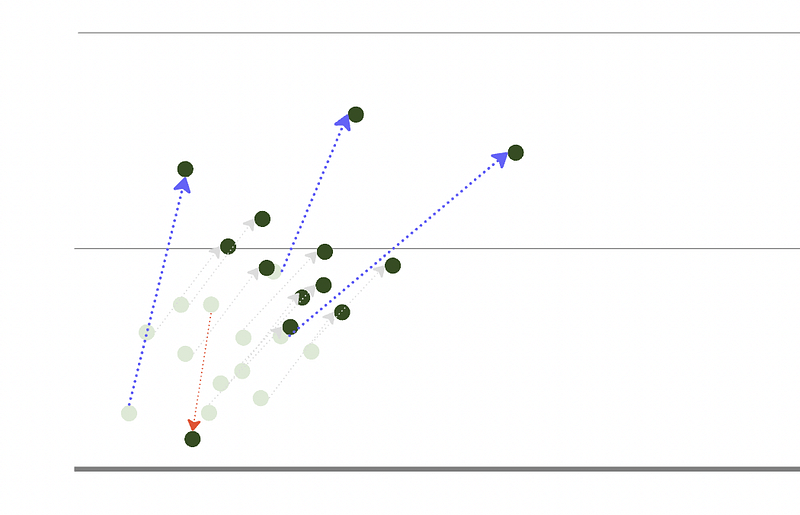
To make it more convenient, you can use the same script to color vectors that differ in the angle of inclination from the total mass by more than the median value of the vector in this mass.
Thus, the graph shows that 4 special cases strayed from the group and improved / worsened more than the rest did.
And since we store a page+request link for each of these points, we can easily check what changes happened to these pages during this series. And understand how they could lead to certain results.
Conclusion
First of all, in conclusion, I would like to list a few remarks that you may have thought about while reading this article:
- For easier understanding and quick preparation of screenshots, the article provides sample data, in real conditions, the clouds will be less obvious and Google Sheets alone will be difficult to manage. It is better to turn this approach armed with an analyst and a python in order to correctly identify pages by points and immediately build motion vectors from series to series, which Google Sheets can only do with the help of tweaks.
- On real data, the model shows a slightly larger spread in degrees of vectors, but it is still not difficult to calculate special cases by an indicator that differs from the median in increments of 15–20%.
- There can be more groups of pages, it is also better to mark them in a data set, and not according to the traffic + position pattern since pages from completely different groups can have the same indicators.
This method of analysis is great for evaluating the effectiveness of the SEO strategy as a whole, and/or mass/targeted changes. It allows you to more subtly probe the changes and accumulate a database, insight, and understanding of your product and what actions lead to certain results.
In my future plans, I am exploring the possibilities of automating the collection of all types of data necessary for the report in order to put this process on stream and assemble a convenient dashboard that will allow me to keep my finger on the pulse not only with global changes but on a regular basis.